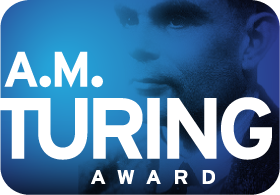
Birth: March 5, 1964 in Paris.
Education: B.Eng. in computer engineering (McGill, 1986); M.Sc. in computer science (McGill, 1988); Ph.D. in computer science (McGill, 1991).
Experience: Massachusetts Institute of Technology: Post-doctoral Fellow, Brain and Cognitive Sciences Dept. (1991-2). Bell Labs: Post-doctoral Fellow, Learning and vision algorithms (1992-3). University of Montreal: Assistant Professor (1993-1997); Associate Professor (1997-2002); Full Professor (2002-Present).
Honors and Awards (selected): Canada Research Chair, Tier 2 (2000); Canada Research Chair, Tier 1 (2006); Government of Quebec, Prix Marie-Victorin (2017); Officer of the Order of Canada (2017); Officer of the Royal Society of Canada (2017); Lifetime Achievement Award, Canadian Artificial Intelligence Association (2018); ACM A.M. Turing Award (2018); Killam Prize in Natural Sciences (2019); Neural Networks Pioneer Award, IEEE Computational Intelligence Society (2019); Fellow of the Royal Society (2020); Princess of Asturias Award for Technical and Scientific Research (2022); Knight of the French Legion of Honor (2023); ACM Fellow (2023).
For conceptual and engineering breakthroughs that have made deep neural networks a critical component of computing.
Yoshua Bengio was born to two college students in Paris, France. His parents had rejected their traditional Moroccan Jewish upbringings to embrace the 1960s counterculture’s focus on personal freedom and social solidarity. He attributes his comfort in following his “scientific intuition” to this upbringing.[1]In search of a more inclusive society, the family moved to Montreal, in the French-speaking Canadian province of Quebec, when Yoshua was twelve years old.
Bengio spent his childhood as a self-described “typical nerd,” bored by high school and reading alone in the library. Like many in his generation he discovered computers during his teenage years, pooling money earned from newspaper delivery with his brother to purchase Atari 800 and Apple II personal computers. This led him to study computer engineering at McGill. Unlike a typical computer science curriculum, this included significant training in physics and continuous mathematics, providing essential mathematical foundations for his later work in machine learning.
After earning his first degree in 1986, Bengio remained at McGill to follow up with a masters’ degree in 1988 and a Ph.D. in computer science in 1991. His study was funded by a graduate scholarship from the Canadian government. He was introduced to the idea of neural networks when reading about massively parallel computation and its application to artificial intelligence. Discovering the work of Geoffey Hinton, his co-awardee, awakened an interest in the question “what is intelligence?” This chimed with his childhood interest in science fiction, in what he called a “watershed moment” for his career. Bengio found a thesis advisor, Renato De Mori, who studied speech recognition and was beginning to transition from classical AI models to statistical approaches.
As a graduate student he was able to attend conferences and workshops to participate in the tight-knit but growing community interested in neural networks, meeting what he called the “French mafia of neural nets” including co-awardee Yann LeCun. He describes Hinton and LeCun as his most important career mentors, though he did not start working with Hinton until years later. He first did a one-year postdoc at MIT with Michael I. Jordan which helped him advance his understanding of probabilistic modeling and recurrent neural networks. Then, as a postdoctoral fellow at Bell Labs, he worked with LeCun to apply techniques from his Ph.D. thesis to handwriting analysis. This contributed to a groundbreaking AT&T automatic check processing system, based around an algorithm that read the numbers written by hand on paper checks by combining neural networks with probabilistic models of sequences.
Bengio returned to Montreal in 1993 as a faculty member at its other major university, the University of Montreal. He won rapid promotion, becoming a full professor in 2002. Bengio suggests that Canada’s “socialist” commitment to spreading research funding widely and towards curiosity-driven research explains its willingness to support his work on what was then an unorthodox approach to artificial intelligence. This, he believes, laid the groundwork for Canada’s current strength in machine learning.
In 2000 he made a major contribution to natural language processing with the paper “A Neural Probabilistic Language Model.” Training networks to distinguish meaningful sentences from nonsense was difficult because there are so many different ways to express a single idea, with most combinations of words being meaningless. This causes what the paper calls the “curse of dimensionality,” demanding infeasibly large training sets and producing unworkably complex models. The paper introduced high-dimensional word embeddings as a representation of word meaning, letting networks recognize the similarity between new phrases and those included in their training sets, even when the specific words used are different. The approach has led to a major shift in machine translation and natural language understanding systems over the last decade.
Bengio’s group further improved the performance of machine translation systems by combining neural word embeddings with attention mechanisms. “Attention” is another term borrowed from human cognition. It helps networks to narrow their focus to only the relevant context at each stage of the translation in ways that reflect the context of words, including, for example, what a pronoun or article is referring to.
Together with Ian Goodfellow, one of his Ph.D. students, Bengio developed the concept of “generative adversarial networks.” Whereas most networks were designed to recognize patterns, a generative network learns to generate objects that are difficult to distinguish from those in the training set. The technique is “adversarial” because a network learning to generate plausible fakes can be trained against another network learning to identify fakes, allowing for a dynamic learning process inspired by game theory. The process is often used to facilitate unsupervised learning. It has been widely used to generate images, for example to automatically generate highly realistic photographs of non-existent people or objects for use in video games.
Bengio had been central to the institutional development of machine learning in Canada. In 2004, a program in Neural Computation and Adaptive Perception was funded within the Canadian Institute for Advanced Research (CIFAR). Hinton was its founding director, but Bengio was involved from the beginning as a Fellow of the institute. So was LeCun, with whom Bengio has been codirecting the program (now renamed Learning in Machines and Brains) since 2014. The name reflects its interdisciplinary cognitive science agenda, with a two-way passage of ideas between neuroscience and machine learning.
Thanks in part to Bengio, the Montreal area has become a global hub for work on what Bengio and his co-awardees call “deep learning.” He helped to found Mila, the Montreal Institute for Learning Algorithms (now the Quebec Artificial Intelligence Institute), to bring together researchers from four local institutions. Bengio is its scientific director, overseeing a federally funded center of excellence that co-locates faculty and students from participating institutions on a single campus. It boasts a broad range of partnerships with famous global companies and an increasing number of local machine learning startup firms. As of 2020, Google, Facebook, Microsoft and Samsung had all established satellite labs in Montreal. Bengio himself has co-founded several startup firms, most notably Element AI in 2016 which develops industrial applications for deep learning technology.
Author: Thomas Haigh
[1] Personal details and quotes are from Bengio’s Heidelberg Laureate interview - https://www.youtube.com/watch?v=PHhFI8JexLg.