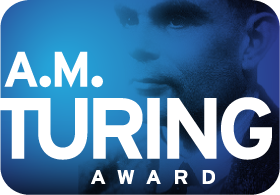
Birth: July 8, 1960 in Soisy-sous-Montmorency, France.
Education: Electrical Engineer Diploma (Ecole Supérieure d'Ingénieurs en Electrotechnique et Electronique, Paris in 1983); PhD in Computer Science (Université Pierre et Marie Curie, 1987).
Experience: University of Toronto: Postdoctoral fellow, 1987-88. Bell Labs Adaptive Systems Research Department: Research Scientist, 1988-1996. AT&T Labs Research: Head, Image Processing Research Department, 1996-2002. NEC Research Institute, Fellow 2002-3. Courant Institute of Mathematical Science, New York University: Professor of Computer Science, 2003-2008; Silver Professor (now of Data Science, Computer Science, Neural Science, and Electrical Engineering, 2008-present. Facebook: Director of AI Research, 2013-18; Vice President and Chief AI Scientist, 2018-present.
Honors and Awards (selected): IEEE Neural Network Pioneer Award (2014); IEEE PAMI Distinguished Researcher Award (2015); Honorary doctorate from Instituto Politécnico Nacional of Mexico (2016); Lovie Lifetime Achievement Award from the International Academy of Digital Arts and Science (2016); Member of the National Academy of Engineering (2017); Pender Award from the University of Pennsylvania (2018); Honorary Doctorate from the Ecole Polytechnique Fédérale de Lausanne (2018); ACM A.M. Turing Award (2018); Fellow of the Association for the Advancement of Artificial Intelligence (2019); Chevalier de la Légion d’Honneur (2020); Princess of Asturias Award for Technical and Scientific Research (2022); Knight of the French Legion of Honor (2023).
For conceptual and engineering breakthroughs that have made deep neural networks a critical component of computing.
Yann LeCun spent his early life in France, growing up in the suburbs of Paris. (His name was originally Le Cun, but he dropped the space after discovering that Americans were confused and treated Le as his middle name). His father was an engineer, whose interests in electronics and mechanics were passed on to Yann during a boyhood of tinkering. As a teenager he enjoyed playing in a band as well as science and engineering. He remained in the region to study, earning the equivalent of a masters’ degree from the École Supérieure d'Ingénieurs en Électrotechnique et Électronique, one of France’s network of competitive and specialized non-university schools established to train to the country’s future elite. His work there focused on microchip design and automation.
LeCun attributes his longstanding interest in machine intelligence to seeing the murderous mainframe HAL, whom he encountered as a young boy in the movie 2001. He began independent research on machine learning as an undergraduate, making it the centerpiece of his Ph.D. work at the Sorbonne Université (then called Université Pierre et Marie Curie). LeCun’s research closely paralleled discoveries made independently by his co-awardee Geoffrey Hinton. Like Hinton he had been drawn to the then-unfashionable neural network approach to artificial intelligence, and like Hinton he discovered the well-publicized limitations of simple neural networks could be overcome by using what was later called the “back-propagation” algorithm to efficiently train “hidden” neurons in intermediate layers between the input and output nodes.
A workshop held in Les Houches in the French Alps in 1985 first brought LeCun into direct contact with the international research community working along these lines. It was there that he met Terry Sejnowski, a close collaborator of Hinton’s whose work on backpropagation was not yet published. A few months later when Hinton was in Paris he introduced himself to LeCun, which led to an invitation to a summer workshop at Carnegie Mellon and a post-doctoral year with Hinton’s new research group in Toronto. This collaboration endured: two decades later, in 2004, he worked with Hinton to establish a program on Neural Computation and Adaptive Perception through the Canadian Institute for Advanced Research (CIFAR). Since 2014 he has co-directed the program, now named Learning in Machines & Brains, with his co-awardee Yoshua Bengio.
At the conclusion of the fellowship, in 1988, LeCun joined the staff of Bell Labs, a renowned center of computer science research. Its Adaptive Systems Research department, headed by Lawrence D. Jackel, focused on machine learning. Jackel was heavily involved in establishing the Neural Networks for Computing workshop series, later run by LeCun and renamed the “Learning Workshop”. It was held annually from 1986 to 2012 at the Snowbird resort in Utah. The invitation-only event brought together an interdisciplinary group of researchers to exchange ideas on the new techniques and learn how to apply them in their own work.
LeCun’s work at Bell Labs focused on the neural network architectures and learning algorithms. His most far-reaching contribution was a new approach, called the “convolutional neural network.” Many networks are designed to recognize visual patterns, but a simple learning model trained to respond to a feature in one location (say the top left of an image) would not respond to the same feature in a different location. The convolutional network is designed so that a filter or detector is swept across the grid of input values. As a result, higher level portions of the network would be alerted to the pattern wherever it occured in the image. This made training faster and reduced the overall size of networks, boosting their performance. This was an extension of LeCun’s earlier achievements, because convolutional networks rely on backpropagation techniques to train their hidden layers.
As well as developing the convolutional approach, LeCun pioneered its application in “graph transformer networks” to recognize printed and handwritten text. This was used in a widely deployed system to read numbers written on checks, produced in the early 1990s in collaboration with Bengio, Leon Bottou and Patrick Haffner. At that time handwriting recognition was enormously challenging, despite an industry-wide push to make it work reliably in “slate” computers (the ancestors of today’s tablet systems). Automated check clearing was an important application, as millions were processed daily. The job required very high accuracy, but unlike general handwriting analysis required only digit recognition, which reduced the number of valid symbols. The technology was licensed by specialist providers of bank systems such as National Cash Register. LeCun suggests that at one point it was reading more than 10% of all the checks written in the US.
Check processing work was carried out in centralized locations, which could be equipped with the powerful computers needed to run neural networks. Increases in computer power made it possible to build more complex networks and deploy convolutional approaches more widely. Today, for example, the technique is used on Android smartphones to power the speech recognition features of the Google Assistant such as real-time transcription, and the camera-based translation features of the translation app.
His other main contribution at Bell Labs was the development of "Optimal Brain Damage" regularization methods. This evocatively named concept identifies ways to simplify neutral networks by removing unnecessary connections. Done properly, this “brain damage” could produce simpler, faster networks that performed as well or better than the full-size version.
In 1996 AT&T, which had failed to establish itself in the computer industry, spun off most of Bell Labs and its telecommunications hardware business into a new company, Lucent Technologies. LeCun stayed behind to run an AT&T Labs group focused on image processing research. His primary accomplishment there was the DjVu image compression technology, developed with Léon Bottou, Patrick Haffner, and Paul G. Howard. High speed Internet access was rare, so as a communications company AT&T’s services would be more valuable if large documents could be downloaded more quickly. LeCun’s algorithm compressed files more effectively than Adobe’s Acrobat software, but lacked the latter’s broad support. It was extensively used by the Internet Archive in the early 2000s.
LeCun left industrial research in 2003, for a faculty position as a professor of computer science at New York University’s Courant Institute of Mathematical Sciences, the leading center for applied mathematical research in the US. It has a strong presence in scientific computation and particular focus on machine learning. He took the opportunity to restore his research focus on neural networks. At NYU LeCun ran the Computational and Biological Learning Lab, which continued his work on algorithms for machine learning and applications for computer vision. He is still at NYU, though as his reputation has grown he has added several new titles and additional appointments. Most notable of these is Silver endowed professorship awarded to LeCun in 2008, funded by a generous bequest from Polaroid co-founder Julius Silver to allow NYU to attract and retain top faculty.
LeCun had retained his love of building things, including hobbies constructing airplanes, electronic musical instrument, and robots. At NYU he combined this interest in robotics with his work on convolutional networks for computer vision to participate in DARPA-sponsored projects for autonomous navigation. His most important institutional initiative was work in 2011 to create the NYU Center for Data Science, which he directed until 2014. The center offers undergraduate and graduate degrees and functions as a focal point for data science initiatives across the university.
By the early 2010s the leading technology companies were scrambling to deploy machine learning systems based on neural networks. Like other leading researchers LeCun was courted by the tech giants, and from December 2013 he was hired by Facebook to create FAIR (Facebook AI Research), which he led until 2018 in New York, sharing his time between NYU and FAIR. That made him the public face of AI at Facebook, broadening his role from a researcher famous within several fields to a tech industry leader frequently discussed in newspapers and magazines. In 2018, he stepped down from the director role and became Facebook’s Chief AI Scientist to focus on strategy and scientific leadership.
Author: Thomas Haigh