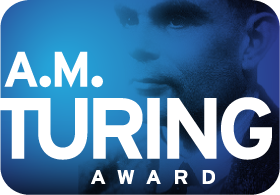
September 4, 1936, Tel Aviv.
B.S., Electrical Engineering (Technion, 1960); M.S., Electronics (Newark College of Engineering, 1961); M.S., Physics (Rutgers University, 1965); Ph.D., Electrical Engineering (Polytechnic Institute of Brooklyn, 1965).
Research Engineer, New York University Medical School (1960–1961); Instructor, Newark College of Engineering (1961); Member of Technical Staff, RCA Research Laboratories, Princeton, New Jersey (1961–1965); Director, Advanced Memory Devices, Electronic Memories, Inc., Hawthorne, California (1966–1969); Assistant Professor of Engineering Systems, UCLA (1969–1970); Associate Professor of Computer Science, UCLA (1970–1976); Director, Cognitive Systems Laboratory, UCLA (from 1978); Professor of Computer Science, UCLA (from 1976—Emeritus since 1994); Professor of Statistics, UCLA (from 1996–Emeritus since 1994); President, Daniel Pearl Foundation (from 2002); International Advisory Board, NGO Monitor (from 2011).
RCA Laboratories Achievement Award (1963); NATO Senior Fellowship in Science (1974); Pattern Recognition Society Award for an Outstanding Contribution (1978); Fellow, IEEE (1988); Fellow, American Association of Artificial Intelligence (1990); Named “The Most Published Scientist in the Artificial Intelligence Journal,” (1991); Member, National Academy of Engineering (1995); UCLA Faculty Research Lecturer of the Year (1996); IJCAI Research Excellence Award (1999); AAAI Classic Paper Award (2000); Lakatos Award, London School of Economics and Political Science (2001); Corresponding Member, Spanish Academy of Engineering (2002); Pekeris Memorial Lecture (2003); ACM Allen Newell Award (2003); Purpose Prize (2006); Honorary Doctorate, University of Toronto (2007); Honorary Doctorate, Chapman University (2008); Benjamin Franklin Medal in Computers and Cognitive Science (2008); Festschrift and Symposium in honor of Judea Pearl (2010); Rumelhart Prize Symposium in honor of Judea Pearl (2011); David E. Rumelhart Prize (2011); IEEE Intelligent Systems’ AI Hall of Fame (2011); ACM Turing Award (2011); Harvey Prize (2012); elected to National Academy of Sciences (2014).
For fundamental contributions to artificial intelligence through the development of a calculus for probabilistic and causal reasoning.
Judea Pearl created the representational and computational foundation for the processing of information under uncertainty.
He is credited with the invention of Bayesian networks, a mathematical formalism for defining complex probability models, as well as the principal algorithms used for inference in these models. This work not only revolutionized the field of artificial intelligence but also became an important tool for many other branches of engineering and the natural sciences. He later created a mathematical framework for causal inference that has had significant impact in the social sciences.
Judea Pearl was born on September 4, 1936, in Tel Aviv, which was at that time administered under the British Mandate for Palestine. He grew up in Bnei Brak, a Biblical town his grandfather went to reestablish in 1924.
Pearl discusses the influence of his inspirational high school teachers in Tel Aviv. |
In 1956, after serving in the Israeli army and joining a Kibbutz, Judea decided to study engineering. He attended the Technion, where he met his wife, Ruth, and received a B.S. degree in Electrical Engineering in 1960. Recalling the Technion faculty members in a 2012 interview in the Technion Magazine, he emphasized the thrill of discovery:
Professor Franz Olendorf always spoke as if he was personally present in Cavendish laboratory, where the electron was discovered, Professor Abraham Ginzburg made us feel the winds blowing in our face as we travelled along those line integrals in the complex plane. And Professor Amiram Ron gave us the feeling that there is still something we can add to Maxwell’s theory of electromagnetic waves.
Judea then went to the United States for graduate study, receiving an M.S. in Electronics from Newark College of Engineering in 1961, an M.S. in Physics from Rutgers University in 1965, and a Ph.D. in electrical engineering from the Polytechnic Institute of Brooklyn in the same year. The title of his Ph.D. thesis was “Vortex Theory of Superconductive Memories;” the term “Pearl vortex” has become popular among physicists to describe the type of superconducting current he studied. He worked at RCA Research Laboratories in Princeton, New Jersey on superconductive parametric amplifiers and storage devices, and at Electronic Memories, Inc. in Hawthorne, California on advanced memory systems. Despite the apparent focus on physical devices, Pearl reports being motivated even then by potential applications to intelligent systems.
When industrial research on magnetic and superconducting memories was curtailed by the advent of large-scale semiconductor memories, Pearl decided to move into academia to pursue his long-term interest in logic and reasoning. In 1969, he joined the faculty of the University of California, Los Angeles, initially in Engineering Systems, and in 1970 he received tenure in the newly formed Computer Science Department. In 1976 he was promoted to full professor. In 1978 he founded the Cognitive Systems Laboratory – a title that emphasized his desire to understand human cognition. The laboratory’s research facility was Pearl’s office, on the door of which hung a permanent sign reading, “Don’t knock. Experiments in Progress.”
Pearl explains that free will is an illusion that might need to be programmed into robots. |
Pearl’s reputation in computer science was established initially not in probabilistic reasoning –a highly controversial topic at that time – but in combinatorial search. A series of journal papers beginning in 1980 culminated in the publication of the book, Heuristics: Intelligent Search Strategies for Computer Problem Solving, [6] in 1984. This work included many new results on traditional search algorithms such as A*, and on game-playing algorithms, raising AI research to a new level of rigor and depth. It also set out new ideas on how admissible heuristics might be derived automatically from relaxed problem definitions, an approach that has led to dramatic advances in planning systems.
Pearl’s book on heuristic search explained the tradeoff between rigor of static evaluation and depth of search. |
Despite the book’s formal style, it drew its inspiration from, as Pearl said, “the ever-amazing observation of how much people can accomplish with that simplistic, unreliable information source known as intuition.” Ira Pohl wrote in 2011 that “The impact of Pearl’s monograph was transformative … [The book] was a tour de force summarizing the work of three decades.”
Soon after arriving at UCLA, Pearl began teaching courses on probability and decision theory, which was a rarity in computer science departments at that time. Probabilistic methods had been tried in the 1960s and found wanting; a system for estimating the probability of a disease given n possible symptoms was thought to require a set of probability parameters whose size is exponential in n. The 1970s, on the other hand, saw the rise of knowledge-based systems, based primarily on logical rules or on rules augmented with “certainty factors.”
Pearl believed that sound probabilistic analysis of a problem would give intuitively correct results, even in those cases where rule-based systems behaved incorrectly. One such case had to do with the ability to reason both causally (from cause to effect) and diagnostically (from effect to cause). “If you used diagnostic rules, you could not do prediction, and if you used predictive rules you could not reason diagnostically, and if you used both, you ran into positive-feedback instabilities, something we never encountered in probability theory.” Another case concerned the “explaining-away” phenomenon, whereby the degree of belief in any cause of a given effect is increased when the effect is observed, but then decreases when some other cause is found to be responsible for the observed effect. Rule-based systems could not exhibit the explaining-away phenomenon, whereas it happens automatically in probabilistic analysis.
In addition to these basic qualitative questions, Pearl was motivated by David Rumelhart’s 1976 paper on reading comprehension. As he wrote later in his 1988 book,
In this paper, Rumelhart presented compelling evidence that text comprehension must be a distributed process that combines both top-down and bottom-up inferences. Strangely, this dual mode of inference, so characteristic of Bayesian analysis, did not match the capabilities of either the “certainty factors” calculus or the inference networks of PROSPECTOR[1]−the two major contenders for uncertainty management in the 1970s. I thus began to explore the possibility of achieving distributed computation in a “pure” Bayesian framework.
Pearl realized that the concept of conditional independence would be the key to constructing complex probability models with polynomially many parameters and to organizing distributed probability computations. The paper “Reverend Bayes on Inference Engines: A Distributed Hierarchical Approach”[8] introduced probability models defined by directed acyclic graphs and derived an exact, distributed, asynchronous, linear-time inference algorithm for trees – an algorithm we now call belief propagation, the basis for turbocodes.
Pearl invented Baysesian networks around conditional independence and belief propagation. |
There followed a period of remarkable creative output for Pearl, with more than 50 papers covering exact inference for general graphs, approximate inference algorithms using Markov chain Monte Carlo, conditional independence properties, learning algorithms, and more, leading up to the publication of Probabilistic Reasoning in Intelligent Systems[15] in 1988. This monumental work combined Pearl’s philosophy, his theories of human cognition, and all his technical material into a persuasive whole that sparked a revolution in the field of artificial intelligence. Within just a few years, leading researchers from both the logical and the neural-network camps within AI had adopted a probabilistic – often called simply the modern – approach to AI.
Pearl’s Bayesian networks provided a syntax and a calculus for multivariate probability models, in much the same way that George Boole provided a syntax and a calculus for logical models. Theoretical and algorithmic questions associated with Bayesian networks form a significant part of the modern research agenda for machine learning and statistics, Their use has also permeated other areas, such as natural language processing, computer vision, robotics, computational biology, and cognitive science. As of 2012, some 50,000 publications have appeared with Bayesian networks as a primary focus.
Even while developing the theory and technology of Bayesian probability networks, Pearl suspected that a different approach was needed to address the issue of causality, which had been one of his concerns for many years. In his 2000 book on Causality [20], he described his early interest as follows:
I got my first hint of the dark world of causality during my junior year of high school. My science teacher, Dr. Feuchtwanger, introduced us to the study of logic by discussing the 19th century finding that more people died from smallpox inoculations than from smallpox itself. Some people used this information to argue that inoculation was harmful when, in fact, the data proved the opposite, that inoculation was saving lives by eradicating smallpox.
And here is where logic comes in,” concluded Dr. Feuchtwanger, “To protect us from cause-effect fallacies of this sort.” We were all enchanted by the marvels of logic, even though Dr. Feuchtwanger never actually showed us how logic protects us from such fallacies.
It doesn’t, I realized years later as an artificial intelligence researcher. Neither logic, nor any branch of mathematics had developed adequate tools for managing problems, such as the smallpox inoculations, involving cause-effect relationships.
A Bayesian network such as Smoking --> Cancer fails to capture causal information; indeed, it is mathematically equivalent to the network Cancer --> Smoking. The key characteristic of a causal network is the way in which it captures the potential effect of exogenous intervention. In a causal network X --> Y, intervening to set the value of Y should leave one’s prior belief in X unchanged and simply breaks the link from X to Y; thus, Smoking --> Cancer as a causal network captures our beliefs about how the world works (inducing cancer in a subject does not change one’s belief in whether the subject is a smoker), whereas Cancer --> Smoking does not (inducing a subject to smoke does change one’s belief that the subject will develop cancer).
Pearl explains how his newer work on causality led him to reconsider his previous beliefs. |
This simple analysis, which Pearl calls the do-calculus, leads to a complete mathematical framework for formulating causal models and for analyzing data to determine causal relationships. This work has overturned the long-held belief in statistics that causality can be determined only from controlled random trials – which are impossible in areas such as the biological and social sciences. Referring to this work, Phil Dawid (Professor of Statistics at Cambridge) remarks that Pearl is “the most original and influential thinker in statistics today.” Chris Winship (Professor of Sociology at Harvard) writes that, “Social science will be forever in his debt.”
Pearl reflects on the importance of rebellion to science. |
In 2010 a Symposium was held at UCLA in Pearl’s honor, and a Festschrift was published containing papers in all the areas covered by his research. The volume also contains reminiscences from former students and other researchers in the field. Ed Purcell, Pearl’s first PhD student, wrote, “In class I was immediately impressed and enchanted by Judea’s knowledge, intelligence, brilliance, warmth and humor. His teaching style was engaging, interactive, informative and fun.” Hector Geffner, a PhD student in the late 1980s, wrote, “He was humble, fun, unassuming, respectful, intelligent, enthusiastic, full of life, very easy to get along with, and driven by a pure and uncorrupted passion for understanding.” Nils Nilsson, former professor and Chair of the Computer Science Department at Stanford and an AI pioneer, described Pearl as “a towering figure in our field.”
Pearl’s outside interests include music (several early conferences were entertained by his impromptu piano renditions and very realistic trumpet imitations), philosophy, and early books – particularly the great works of science throughout history, of which he possesses several first editions. Judea and Ruth Pearl had three children, Tamara, Michelle, and Daniel. Since Daniel’s kidnap and murder in Pakistan in 2002, Professor Pearl has devoted a significant fraction of his time and energy to the Daniel Pearl Foundation, which he and his wife founded to promote Daniel’s values of “uncompromised objectivity and integrity; insightful and unconventional perspective; tolerance and respect for people of all cultures; unshaken belief in the effectiveness of education and communication; and the love of music, humor, and friendship.”
Pearl will donate a major portion of the Turing Prize money to support the projects of the Daniel Pearl Foundation and another portion to promote the introduction of causal inference in statistics education.
Author: Stuart J. Russell
[1] An expert system that finds ore deposits from geological information; created in the 1970s by Richard Duda, Peter Hart, and others at Stanford Research Institute (SRI).