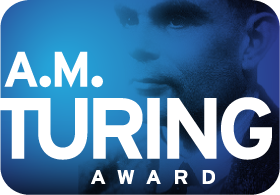
Birth: December 6, 1947 in Wimbledon, England.
Education: Bachelor’s degree in Experimental Psychology (Cambridge University, 1970); Ph.D. in Artificial Intelligence (University of Edinburgh, 1978).
Experience: Research Fellow Cognitive Studies Program, Sussex University (1976-78); Visiting Scholar Program in Cognitive Science, University of California, San Diego (1978-1980); Scientific Officer, MRC Applied Psychology Unit, Cambridge, England (1980-1982); Visiting Assistant Professor Psychology Department, University of California, San Diego (1982); Assistant Professor then Associate Professor Computer Science Department, Carnegie-Mellon University (1982-1987); Professor, Computer Science Department, University of Toronto (1987-1998); Founding Director of the Gatsby Computational Neuroscience Unit University College London (1998-2001); Professor, Computer Science Department, University of Toronto (2001-2006); ); University Professor, Computer Science Department, University of Toronto (2006-2014); Distinguished Researcher, Google (2013-2016); Vice President & Engineering Fellow, Google (2016-2023); Chief Scientific Advisor, Vector Institute (2017-Present).
Honors and Awards (selected): IEEE Signal Processing Society Senior Award (1990); Fellow, Association for the Advancement of Artificial Intelligence (1991); IEEE Neural Networks Pioneer Award (1992); Fellow of the Royal Society of Canada (1996); ITAC/NSERC award for academic excellence (1998); Fellow of the Royal Society (1998); David E. Rumelhart Prize (2001); Honorary Doctorate from the University of Edinburgh (2001); Fellow of the Cognitive Science Society (2003); IJCAI Research Excellence Award (2005); Gerhard Herzberg Canada Gold Medal (2010); Honorary Doctorate from the University of Sussex (2011); Killam Prize in Engineering (2012); Honorary Doctorate from the University of Sherbrooke (2013); IEEE Frank Rosenblatt Medal (2014); Distinguished Fellow, Canadian Institute for Advanced Research (2014); IEEE/RSE James Clerk Maxwell Gold Medal (2016); NEC C&C Award (2016); BBVA Foundation Frontiers of Knowledge Award (2017); Companion of the Order of Canada (2018); ACM A. M. Turing Award (2018);Honda Prize (2019); Dickinson Prize in Science (2021); Princess of Asturias Award for Technical and Scientific Research (2022); ACM Fellow (2023); Nobel Prize in Physics (2024).
For conceptual and engineering breakthroughs that have made deep neural networks a critical component of computing.
When Geoffrey Everest Hinton decided to study science he was following in the tradition of ancestors such as George Boole, the Victorian logician whose work underpins the study of computer science and probability. Geoffrey’s great grandfather, the mathematician and bigamist Charles Hinton, coined the word “tesseract” and popularized the idea of higher dimensions, while his father, Howard Everest Hinton, was a distinguished entomologist. Their shared middle name, Everest, celebrates a relative after whom the mountain was also named (to commemorate his service as Surveyor General of India).
Having begun his time at Cambridge University with plans to study physiology and physics, before dabbling in philosophy on his way to receiving a degree in experimental psychology in 1970, Hinton concluded that none of these sciences had yet done much to explain human thought. He made a brief career shift into carpentry, in search of more tangible satisfactions, before being drawn back to academia in 1972 by the promise of artificial intelligence, which he studied at the University of Edinburgh.
By the mid-1970s a perception of unfulfilled promises had reduced funding and enthusiasm for artificial intelligence research in the UK. Hinton was drawn to a particularly unfashionable area: the development of networks of simulated neural nodes to mimic the capabilities of human thought. This willingness to ignore conventional wisdom was to characterize his career. As he put it, “If you think it’s a really good idea and other people tell you it’s complete nonsense then you know you are really onto something.”[1]
The relationship of computers to brains had captivated many computer pioneers of the 1940s, including John von Neumann who used biological terms such as “memory,” “organ” and “neuron” when first describing the crucial architectural concepts of modern computing in the “First Draft of a Report on the EDVAC.” This was influenced by the emerging cybernetics movement, particularly the efforts of Warren McCulloch and Walter Pitts to equate networks of stylized neurons with statements in boolean logic. That inspired the idea that similar networks might, like human brains, be able to learn to recognize objects or carry out other tasks. Interest in this approach had declined after Turing Award winner Marvin Minsky, working with Seymour Papert, demonstrated that a heavily promoted class of neural networks, in which inputs were connected directly to outputs, had severe limits on its capabilities.
Graduating in 1978, Hinton followed in the footsteps of many of his forebears by seeking opportunities in the United States. He joined a group of cognitive psychologists as a Sloan Foundation postdoctoral researcher at the University of California, San Diego. Their work on neural networks drew on a broad shift in the decades after the Second World War towards Bayesian approaches to statistics, which treat probabilities as degrees of belief, updating estimates as data accumulates.
Most work on neural networks relies on what is now called a “supervised learning” approach, exposing an initially random network configuration to a “training set” of input data. Its initial responses would have no systematic relationship to the features of the input data, but the algorithm would reconfigure the network as each guess was scored against the labels provided. Thus, for example, a network trained on a large set of photographs of different species of fish might develop a reliable ability to recognize whether a new picture showed a carp or a tuna. This required a learning algorithm to automatically reconfigure the network to identify “features” in the input data that correlated with correct outputs.
Working with David Rumelhart and Ronald J. Williams, Hinton popularized what they termed a “back-propagation” algorithm in a pair of landmark papers published in 1986. The term reflected a phase in which the algorithm propagated measures of the errors produced by the network’s guesses backwards through its neurons, starting with those directly connected to the outputs. This allowed networks with intermediate “hidden” neurons between input and output layers to learn efficiently, overcoming the limitations noted by Minsky and Papert.
Their paper describes the use of the technique to perform tasks including logical and arithmetic operations, shape recognition, and sequence generation. Others, including Paul J. Werbos, had worked independently along similar lines without much impact. Hinton attributes the impact of his work with Rumelhart and Williams to the publication of a summary of their work in Nature, and the efforts they made to provide compelling demonstrations of the power of the new approach. Their findings began to revive enthusiasm for the neural network approach, which has increasingly challenged other approaches to AI such as the symbol processing work of Turing Award winners John McCarthy and Marvin Minsky and the rule-based expert systems championed by Edward Feigenbaum.
By the time the papers with Rumelhart and William were published, Hinton had begun his first faculty position, in Carnegie Mellon’s computer science department. This was one of the leading computer science programs, with a particular focus on artificial intelligence going back to the work of Herb Simon and Allen Newell in the 1950s. But after five years there Hinton left the United States in part because of his opposition to the “Star Wars” missile defense initiative. The Defense Advanced Research Projects Agency was a major sponsor of work on AI, including Carnegie Mellon projects on speech recognition, computer vision, and autonomous vehicles. Hinton first became a fellow of the Canadian Institute for Advanced Research (CIFAR) and moved to the Department of Computer Science at the University of Toronto. He spent three years from 1998 until 2001 setting up the Gatsby Computational Neuroscience Unit at University College London and then returned to Toronto.
Hinton’s research group in Toronto made a string of advances in what came to be known as “deep learning”, named as such because it relied on neural networks with multiple layers of hidden neurons to extract higher level features from input data. Hinton, working with David Ackley and Terry Sejnowski, had previously introduced a class of network known as the Boltzmann machine, which in a restricted form was particularly well-suited to this layered approach. His ongoing work to develop machine learning algorithms spanned a broad range of approaches to improve the power and efficiency of systems for probabilistic inference. In particular, his joint work with Radford Neal and Richard Zemel in the early 1990s introduced variational methods to the machine learning community.
Hinton carried this work out with dozens of dozens of Ph.D. students and post-doctoral collaborators, many of whom went on to distinguished careers in their own right. He shared the Turing award with one of them, Yann LeCun, who spent 1987-88 as a post-doctoral fellow in Toronto after Hinton served as the external examiner on his Ph.D. in Paris. From 2004 until 2013 he was the director of the program on "Neural Computation and Adaptive Perception" funded by the Canadian Institute for Advanced Research. That program included LeCun and his other coawardee, Yoshua Bengio. The three met regularly to share ideas as part of a small group. Hinton has advocated for the importance of senior researchers continuing to do hands-on programming work to effectively supervise student teams.
Hinton has long been recognized as a leading researcher in his field, receiving his first honorary doctorate from the University of Edinburgh in 2001, three years after he became a fellow of the Royal Society. In the 2010s his career began to shift from academia to practice as the group’s breakthroughs underpinned new capabilities for object classification and speech recognition appearing in widely used systems produced by cloud computing companies such as Google and Facebook. Their potential was vividly demonstrated in 2012 when a program developed by Hinton with his students Alex Krizhevsky and Ilya Sutskever greatly outperformed all other entrants to ImageNet, an image recognition competition involving a thousand different object types. It used graphics processor chips to run code combining several of the group’s techniques in a network of “60 million parameters and 650,000 neurons” composed of “five convolutional layers, some of which are followed by max-pooling layers, and three globally-connected layers with a final 1000-way softmax.”[2] The “convolutional layers” were an approach originally conceived of by LeCun, to which Hinton’s team had made substantial improvements.
This success prompted Google to acquire a company, DDNresearch, founded by Hinton and the two students to commercialize their achievements. The system allowed Google to greatly improve its automatic classification of photographs. Following the acquisition, Hinton became a vice president and engineering fellow at Google. In 2014 he retired from teaching at the university to establish a Toronto branch of Google Brain. Since 2017, he has held a volunteer position as chief scientific advisor to Toronto’s Vector Institute for the application of machine learning in Canadian health care and other industries. Hinton thinks that in the future teaching people how to train computers to perform tasks will be at least as important as teaching them how to program computers.
Hinton has been increasingly vocal in advocating for his long-standing belief in the potential of “unsupervised” training systems, in which the learning algorithm attempts to identify features without being provided large numbers of labelled examples. As well as being useful these unsupervised learning methods have, Hinton believes, brought us closer to understanding the learning mechanisms used by human brains.
The 2024 Nobel Prize in Physics was shared between Hinton and John J. Hopfield. This made Hinton only the second person, after Herb Simon in 1978, to have received both a Turing award and a Nobel Prize. Hinton makes no claim to be a physicist, having abandoned the field early in his undergraduate studies, but the prize committee cited his use of tools from statistical physics in the mid-1980s when creating the Boltzman machine. More generally the decision, together with the award the same year of the Nobel Prize in Chemistry to a group including machine learning specialists Demis Hassabis and John Jumper, reflected a new recognition of the importance of computational methods in general, and neural networks in particular, to scientific research across a range of disciplines.
By then, Hinton had already begun to distance himself from the results of his inventions. In 2023 he resigned from Google and issued a dramatic warning that commercial use of generative AI systems posed multiple serious threats such as computer-generated misinformation and the destabilization of the job market. Longer term, he cautioned, autonomous AI systems might pose a serious threat to humanity. According to a New York Times report, Hinton felt that "a part of him... now regrets his life's work." [3]
Author: Thomas Haigh
[3] Metz, Cade. "‘The Godfather of A.I.’ Leaves Google and Warns of Danger Ahead," New York Times, May 1, 2023.